Top 8 Global Data Companies in Solar
Navigating Solar Data Analytics: A Comprehensive Guide
In the midst of a solar energy boom projected to significantly increase global capacity, the imperative for digitalization and automation within the industry has never been more apparent. With forecasts suggesting a tripling of renewable energy capacity in the US and a doubling of solar capacity in Europe over the next decade (IEA, 2023), efficient management of solar installations at unprecedented scales demands powerful analytical tools.
As the solar industry becomes increasingly digitized, stakeholders are turning to various forms of data analysis to optimize performance and ensure reliable operation of solar assets. Amidst this landscape, it’s crucial to understand the nuances between monitoring, analytics, and predictive analytics, and discern their respective applications at different stages of solar asset management.
Selecting the Right Approach at Each Stage
- Early Detection and Prevention:
- Diagnostic Analytics: Offers historical and real-time data analysis, aiding in understanding current and past performance.
- Predictive Analytics: Leverages historical data and external insights to anticipate future issues, enabling proactive measures to prevent downtime and optimize efficiency.
- Comprehensive System Optimization:
- Advanced Analytics: Provides a spectrum of insights, from hindsight and descriptive analytics to foresight-driven predictive and prescriptive analytics.
SmartHelio’s Positioning: Our expertise in solar asset management and predictive analytics enables us to offer groundbreaking solutions. By leveraging vast real-world data and advanced AI algorithms, we empower solar operators with actionable intelligence, focusing on early symptom detection and preemptive maintenance.
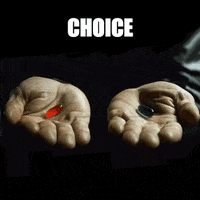
Bring speed to your O&M operations
Impact validated by SmartHelio’s clients using predictive analytics:
Using our solution, clients have experienced on average:
- Portfolio level increase in performance: 6.39%
- Plant with Max performance increase: 8.77%
- Plant with least performance increase: 3.98%
- Revenue gain: EUR 4644 per MW per Year
- Response time improvement (within one month): 1.86 times faster
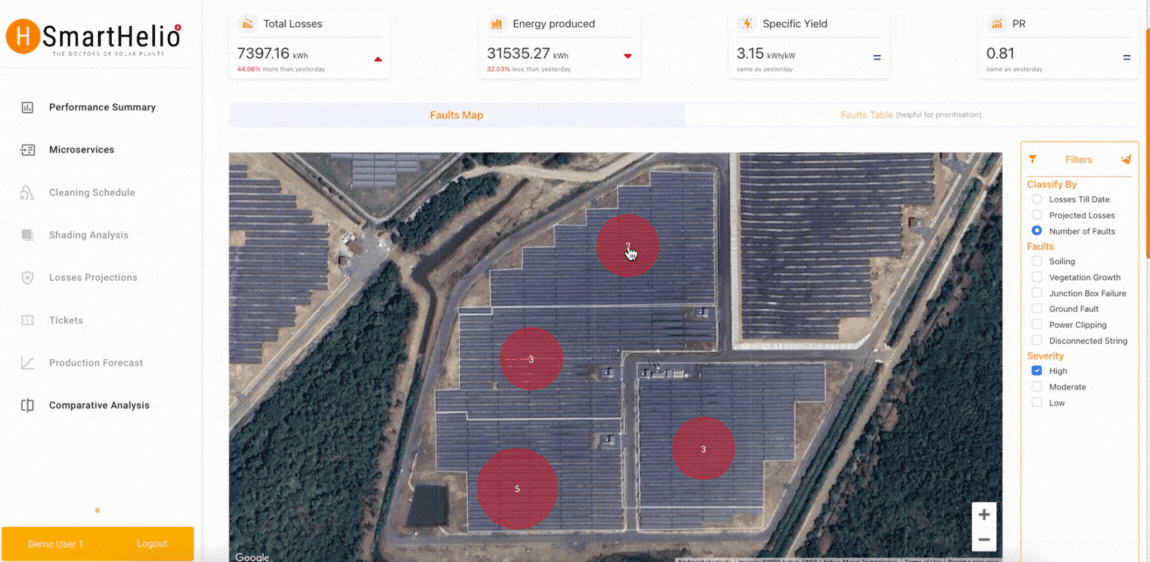
What makes SmartHelio different?
- Vertical integration: After 10+ years of R&D as an EPFL spin off, we specifically focus on automation of fault detection, classification and prediction. Our system has an easy and seamless integration via API to the rest of the client’s value chain.
- Failure prediction: Based on early signatures (“symptoms”), our algorithms enable the prediction of component failures with accuracy over 90%.
- Automatic prescription: Depending on identified faults and priorities, the system automatically prescribes timely actions. This can be further personalized based on client’s O&M strategy, know-how and habits using feedback loop.
- Faults from day 1: Our physics-informed AI algorithms were developed based on pattern recognition (IV fault signatures) over 5bn data points from PV plants across the globe. No long-term data training required, you get results on faults classification from day 1 of onboarding.
- Data privacy: While we start with the open, expansive foundation model, we customize it to suit specific datasets. The data remains the sole property of the client and remains confined within the client’s server.
- Environmentally conscious: ESG certifications to testify the positive impact on the planet and its people. SmartHelio is B-Corp certified and was announced as “Best for the World” environmental category in 2022.
Curious to know more?
Contact SmartHelio’s team of solar data experts. Let’s bring speed and automation to your operations, to maximize your PV performance.
Digital Twins: Unlocking the Power of AI & ML in Solar
Digital Twins: Unlocking the Power of AI & ML in Solar
RECORDING AVAILABLE
The integration of artificial intelligence and machine learning into solar O&M isn’t just hype – it’s a reality that’s enhancing efficiency and sustainability. With computer-simulated technology at the forefront, the solar sector is witnessing a paradigm shift in asset performance optimization, cost reduction, and emission mitigation. This webinar invites you to explore how Digital Twin technology is evolving and the opportunities it presents for asset managers to excel in both operational efficiency and profitability.
What to expect
- 💡 Advanced Predictive Analytics: Harness physics-informed AI to predict and prevent failures in real-time, boosting performance by up to 10% and optimizing O&M resources by 30%.
- 🚀 Autopilot Software: Utilize 50+ physics-based algorithms for accurate fault prediction, with immediate results and no long-term data training required.
- 💬 ChatGPT Integration: Simplify data analysis and decision-making through interactive Q&A sessions and concise report generation, ensuring actionable insights for stakeholders.
Get access to recording
Free Diagnostics
Free Diagnostics
Diagnose the root causes of your PV plant’s underperformance.
SmartHelio has launched a complimentary service to quickly help you identify the root causes of the underperformance of your solar plant. In only two steps, receive a detailed diagnostics with root causes and a list of actions to be taken. Why would you sign up for this service?
- Comprehensive Root Cause Analysis: Benefit from our extensive portfolio of over 50 fault diagnostics, ensuring a thorough examination of your PV plant’s performance.
- Loss Quantification: Gain insights into the quantifiable losses associated with different faults and issues affecting your solar plant.
- Forecasted Impact Assessment: Anticipate the impact of existing root causes, enabling proactive decision-making for optimal performance.
What to expect from this service?
- Expected kWh generation.
- Waterfall/Loss Donut.
- Heatmap of I, V, and SY with generic explanation.
- Possible addition of explanation of the observations in particular heatmaps.
- Losses till date of all recoverable faults.
- Expected per MW annual loss from the identified recoverable losses.
What is Free Diagnostics solving?
There is still a lot of intervention to identify the root cause and we want to help the industry to save time and reduce underperformance to produce more renewable energy. This option is created to relieve the immediate underperformance issues
Powered by our proprietary physics-based AI algorithms, we are aiming to make our technology accessible to the industry and together produce more renewable energy.
Who is it for?
- Asset Managers: Streamline decision-making processes and enhance overall plant performance.
- Performance Teams: Receive targeted insights to optimize efficiency and output.
- O&M Managers: Identify and address underperformance issues promptly, minimizing downtime.
- Control Room & Monitoring: Bolster your monitoring efforts with precise diagnostic information for proactive plant management.
Apply now
January 3, 2024
From monitoring to prevention: A closer look at the solar advanced analytics spectrum
From monitoring to prevention: A closer look at the solar advanced analytics spectrum
Central monitoring systems (CMS) are crucial for real-time data collection but often lack comprehensive data processing and actionable insights, leading to their integration with advanced analytics tools. With the number and variety of tools growing, it’s vital to understand and distinguish the four main analytics categories: descriptive, diagnostic, predictive, and prescriptive.
In this white paper, we debunk misconceptions about the evolving advanced analytics spectrum, explore the distinctions among the four branches, and hone in on the revolutionary potential of predictive and prescriptive analytics. We delve into what differentiates them, the technology driving them, and their impact on the increasingly digital and automated solar industry through a real-world case study that compares diagnostic and predictive analytics.
In a nutshell
- 🌟 The Evolution of Advanced Analytics: Understand the branches of advanced analytics and the unique position of predictive analytics, equipped with machine learning algorithms for early fault detection.
- 📈 Maximizing Solar ROI: Learn how predictive and prescriptive analytics reduces losses and cuts maintenance costs, enhancing solar investment profitability.
- 💡 Real-World Case Study: Explore case studies comparing predictive analytics to diagnostic analytics, highlighting early fault prediction and efficient resolution.
Who is this for?
- 🔧 Asset Managers and O&M Professionals: If you're involved in solar asset management or operations, this white paper will equip you with the knowledge to make data-driven decisions that can lead to more efficient maintenance and increased performance.
- 💰 Investors and Stakeholders: For investors and stakeholders, understanding how predictive analytics can minimize losses and reduce maintenance costs is crucial to maximize your return on investment.
- 💫 Industry Leaders and CXOs: The solar industry is evolving at an unprecedented pace. Don't miss the opportunity to stay informed about the latest innovations in solar advanced analytics and asset management.
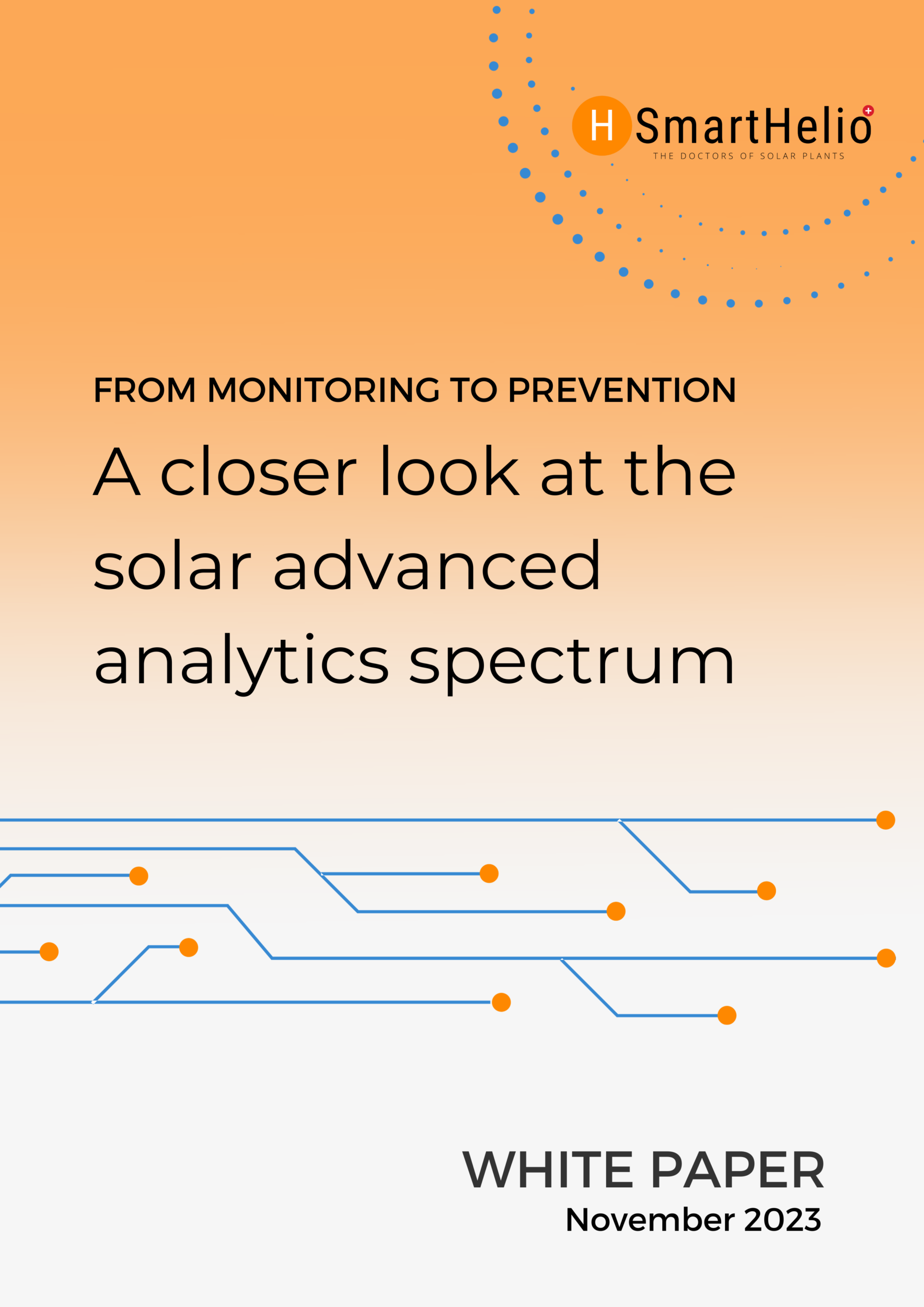
Get access
From monitoring to prevention: A closer look at the solar advanced analytics spectrum
From monitoring to prevention: A closer look at the solar advanced analytics spectrum
RECORDING AVAILABLE
In this engaging webinar to uncover the power of predictive and prescriptive analytics in the rapidly expanding solar industry. In this discussion, our speakers demystify the evolving landscape of advanced analytics, share real-world success stories showcasing the benefits of predictive analytics, and provide valuable insights into the digitalization and automation shaping the solar industry’s future.
What to expect
- 🌟 The Evolution of Advanced Analytics: Discover the main branches of advanced analytics and see how predictive analytics is at the frontier with machine learning algorithms that predict faults and forecast performance.
- 💰 Maximizing Performance with Predictive Analytics: Learn how predictive & prescriptive analytics can significantly reduce losses and maintenance costs and increase the lifespan of solar assets.
- 💡 Real-World Fault Prediction: Explore real-world case studies that exemplify the tangible benefits of predictive analytics in comparison to diagnostic analytics, from fault prediction to swift, targeted fault resolution.
Why you should watch
- 💫 Stay Ahead of the Curve: Don't miss the opportunity to stay informed about the latest innovations in solar advanced analytics and asset management.
- 🔧 Optimize Your Operations: This webinar will equip you with the knowledge to upgrade your O&M to be more proactive.
- 🎙️ Meet the Experts and Join the Discussion: Our experts and leaders in the field are ready to answer your questions and provide insights based on their vast experience.
Get access to recording
Is Your Data Clean? Data Sanitization for Actionable Analytics
Is Your Data Clean? Data Sanatization for Actionable Analytics
Data is crucial for making informed decisions regarding the O&M of a solar plant. On average, however, up to 20% of pre-analytics data is missing or inaccurate, which only leads to missing or inaccurate knowledge of a plant’s performance. Led by data scientists, this webinar identified common data quality issues felt by asset management teams and presented possible solutions, including SmartHelio’s wholistic approach to data sanitization.
What to expect
- 📊 Common data quality issues like corrupt or missing pyranometer data, misconfigured software, and network connection failure
- 💡 The impacts data quality issues have on solar plant operations such as yielding skewed performance metrics
- 🚀 How missing or inaccurate data can be corrected with SmartHelio’s data sanitization algorithms to achieve sharper, more accurate analytics
Who is this webinar for?
- ➡️ Asset managers
- ➡️ O&M teams
- ➡️ Solar data scientists
- ➡️ Heads of innovation
- ➡️ Directors of digitalization
Get access to recording
Please use the form provided below to fill in your information. Once submitted, we will send you access to the recording.
Protecting PV plants against climate change with Autopilot
During July 2023, the world witnessed unprecedented heatwaves and soaring global temperatures, leaving a profound impact on our planet, and raising concerns about the implications for solar assets. In this context, the renewable energy sector is confronted with significant challenges, particularly impacting solar power production and the longevity of inverters, panels, and Balance of System (BOS) components. With the frequency of extreme weather events on the rise, solar plants face potential performance declines and increased maintenance costs.
This blog article explores the effects of these conditions on solar panels and presents a case study featuring one of our clients. This 25 MW utility solar plant in South France highlights the effectiveness of advanced predictive platforms like SmartHelio Autopilot, and how these technologies empower operators to anticipate and address climate-induced issues, ensuring sustainable and efficient energy generation.
The Adverse Effects of Climate Change
Rising global temperatures, more intense heatwaves, and erratic weather patterns significantly impact solar power production. High temperatures exacerbate soiling on solar panels, reducing energy yield, and necessitating more frequent cleaning interventions. Additionally, increased ambient temperatures lead to decreased current carrying capacity of fuses, resulting in progressive string disconnections, as demonstrated in this case study.
Moreover, extreme weather events like storms, hail, and heavy rainfall can damage panels and BOS components, decreasing their longevity and causing unexpected downtime and maintenance costs. The increased wear and tear on inverters and other vital equipment further heightens the need for proactive maintenance strategies.
The Power of SmartHelio Autopilot
The case study is based on a 25 MW utility solar plant in South France and highlights the value of SmartHelio Autopilot in mitigating adverse effects of climate change on solar plants. Its advanced predictive analytics capabilities enabled the detection of progressive string disconnections and high soiling issues caused by the heatwave, prompting the client to take proactive action. Here‘s how:
Anticipating Climate-Induced Problems
By continuously analysing historical and real-time data, SmartHelio Autopilot accurately predicted the occurrence of string disconnections based on weather forecasts and its impact on the power generation, DC side current, and voltage gradients.
This early detection allowed the client to take preventive measures before any significant losses occurred.
Optimizing Maintenance Strategies
With the knowledge of upcoming rain forecasts, SmartHelio Autopilot assisted in planning cleaning interventions to mitigate the impact of soiling on the plant’s performance.
By optimizing the cleaning schedule, the client effectively maintained panel efficiency while minimizing resource consumption.
Enhancing Component Longevity
By identifying the issues affecting the inverters and promptly resolving the inverter issue, SmartHelio Autopilot contributed to extending the longevity of critical BOS components.
Proactive measures taken in response to the platform’s predictions reduced downtime and minimized unplanned maintenance expenses.
The graph below represents a correlation between the frequency of weekly string disconnections and the corresponding increase in average ambient temperature. Using SmartHelio’s proprietary algorithms based on Physics-Informed AI that considers factors like high temperature, irradiations, and OEM specifications, etc, it was able to predict the number of string disconnections in the upcoming weeks. This helped the client to take action and replace the inverters before they blew.
Conclusion
As the effects of climate change continue to challenge the solar power industry, the role of predictive analytics platforms like SmartHelio Autopilot becomes increasingly crucial. The case study of the 25 MW utility solar plant in South France demonstrated how such advanced tools empower operators to anticipate issues and take proactive actions. By accurately predicting the impact of high temperatures on soiling and fuse burn out, SmartHelio Autopilot enabled the client to safeguard their plant’s performance, maximise energy generation, and reduce maintenance costs.
Embracing advanced predictive platforms like Autopilot equips solar plant operators with the insights needed to navigate the ever-changing climate challenges. Through intelligent analytics and early detection, these platforms pave the way for a resilient and sustainable future in solar energy generation, making the vision of a clean and renewable energy world a tangible reality.
Demystifying predictive analytics and preventive maintenance in the solar industry
“The future depends on what you do today.” – Mahatma Gandhi
The rise of predictive analytics and preventive maintenance in the solar industry has gained significant attention in recent years. Indeed, in a rapidly expanding industry, with the US solar market projected to grow by 20% and Europe’s solar capacity estimated to double by 2030, the urgent need for digitalization and predictive analytics becomes evident to effectively manage and ensure optimal performance of solar installations at scale.
However, amidst this constantly increasing buzz, which recently reached a new high at Intersolar Europe 2023, there are misconceptions that should be addressed to truly understand the essence of these terms and ensure we are all talking about the same thing. At SmartHelio, we are committed to providing accurate and actionable insights to help solar industry stakeholders de-risk investments, maximize performance, and reduce costs. Staying true to our mission, in this article we will demystify predictive analytics and preventive maintenance and shed light on their true meaning.
Understanding Predictive Analytics
Predictive analytics in the solar industry is not only leveraging historical labelled data and advanced algorithms to forecast production and predict major component failures like inverters. It also includes the understanding of the early signs of underperformance based on system behaviour anomalies. Predictive analytics encompasses the analysis of a fault’s behaviour with time and projected losses associated with it which helps in prioritizing the proactive actions to be taken.
By analysing past data, understanding the real-time electrical patterns in combination with weather patterns, solar irradiation, and system parameters, predictive analytics can enable the identification of potential issues before they cause significant performance loss and possible equipment failures. SmartHelio’s Autopilot platform uses physics-informed AI, combining Machine Learning techniques with domain expertise, to deliver reliable and accurate predictions and insights.
“Whereas physics-based models are very good on post failure analysis, with more Machine Learning knowledge in our data warehouse we will then be able to recognise very small deviations at an early stage to have prediction on where your next fault may probably happen”
– David Ebner, Project Engineer at VERBUND
Relying solely on AI for predictive analytics in solar, without considering physics-informed models, falls short in several ways. Depending on historical data alone neglects real-time changes, emerging trends, and unforeseen scenarios. It can lead to incomplete understandings, biased predictions, and inaccurate results. By integrating physics principles into AI models, a more comprehensive and reliable approach to predictive analytics in solar is achieved, debunking other solutions who solely rely on AI whilst neglecting consideration for the underlying physics.
“When trying to overcome these barriers in today’s AI methods researchers turn back to an old principle of technology development – understanding nature and learning from it. They ask, how can we build AI systems that can tap into the knowledge we have about the physics of our world?”
– Olympia Brikis, Head of Physics-Informed AI at Siemens,
‘The hidden Potential of Physics-informed AI’ 2022 Siemens
The Essence of Preventive Maintenance
Preventive maintenance is a critical aspect of ensuring optimal performance and longevity of solar installations. It involves taking proactive measures to prevent equipment failures and mitigate potential risks of underperformance. Preventive maintenance today focuses mainly on regular inspections, servicing, and repairs of the mechanical and electrical infrastructures of a PV plant. However, these regular inspections are often periodic based, not issue focused, or can be investigative in a broad sense only after a failure or underperformance has been identified.
Lacking data-driven intelligence, such interventions are not an efficient but reactive use of resources, and they come at significant cost. For example, preventive maintenance based solely on regular interventions cannot consider data-driven intelligence that may detect component behaviour anomalies. By incorporating real-time data analytics, predictive maintenance can optimize performance by identifying and addressing potential issues before they escalate, ensuring optimised system performance and reducing unnecessary maintenance activities and cost.
How is it working?
In solar preventive maintenance, we can draw a parallel to tachycardia, a condition that signals an impending heart attack. Just as specific heart frequency patterns indicate an issue, regardless of who the person is, anomalies in solar component behaviour, such as inverter shutdown or tracker malfunction, can be detected early.
By recognizing these patterns, proactive measures can be taken to prevent system failures and optimize solar plant performance. Similar to understanding the biology of the heart to be able to detect a heart attack, one must deeply understand the physics of solar to make sense of the electrical patterns.
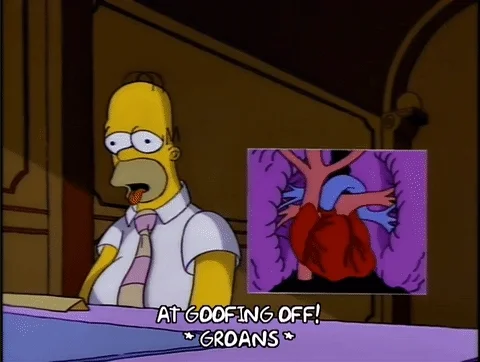
By identifying and addressing issues at an early stage, preventive maintenance minimises downtime, improves energy production, and reduces maintenance costs. SmartHelio adopts a comprehensive preventive maintenance strategy that goes beyond relying solely on SCADA/monitoring alerts. We proactively assess equipment health, provide recommendations based on data-driven insights and prompt preventive maintenance scheduling based on component information analytics developed over 5GW of global assets management.
Monitoring vs. Analytics in Preventive Maintenance
It is important to understand the distinction between monitoring and analytics in the context of preventive maintenance. Monitoring involves real-time data collection and basic alerts that provide immediate information about the current state of the system. Whilst monitoring is essential for detecting immediate issues, it lacks the in-depth analysis and long-term insights that analytics offer.
Analytics, on the other hand, involves advanced data processing, correlation analysis, and predictive modelling in order to provide technical, financial and economical actionable intelligence. It uncovers hidden patterns, identifies trends, and provides actionable insights for preventive maintenance planning. SmartHelio’s Autopilot platform empowers monitoring systems, enabling granular analysis, accurate fault predictions, and data-driven decision-making for efficient preventive maintenance strategies. The plug-in software platform integrates seamlessly with the existing SCADA or CMS monitoring system in order to automate and accelerate accurate fault classification, failure prediction and action prescription.
“Increase PV power generated by the panel is one value. There was a series of ground fault, which may cause over-voltage and even fire risk […] Safety is number one priority for Schneider. We see safety improvement as a second value to (SmartHelio’s) technology”
– Luc Hossenlopp, CTO Digitalisation at Schneider Electric
Differentiating SmartHelio as a Reference in Predictive Analytics
To truly understand the meaning SmartHelio attributes to predictive analytics, let’s explore concrete use cases in action
Early Detection of Declining Panel Efficiency
By analysing historical data and tracking performance trends, SmartHelio’s predictive analytics algorithms could identify gradual reductions in panel efficiency. In our latest finding from a 75MW site in Italy, our software detected 0.78% higher degradation at the end of 3rd year since commissioning.
This early detection enabled timely interventions and warranty claim coordination with the OEM to ensure optimal performance and maximize energy production.
Predicting Potential Faults in the Inverter System
Leveraging physics-informed ML models, SmartHelio’s analytics platform identified patterns and anomalies in inverter system data. Overheating issues were detected as the probable cause. Our predictive intervention avoided significant resources to find the root cause & fix the failure of this 1.75MW inverter.
By detecting potential faults before they occur, our platform enabled proactive, targeted maintenance, reducing downtime and avoiding costly system failure losses (~EUR 787 per day).
Optimising Trimming Schedules Based on Vegetation Rate Projection
SmartHelio’s advanced analytics considered utilized advanced pattern recognition algorithms to detect evolution of horizons profile. Supervised ML models then identified pattern of vegetation, and optimized trimming interventions based on projected vegetation growth trends which was forecasted to be impact the performance by 0.018% per week in this 20MW plant.
By intelligently planning trimming operations, solar assets could maintain optimal performance while minimizing unnecessary trimming costs.
These examples highlight SmartHelio’s ability to deliver accurate and actionable insights, empowering solar industry stakeholders to make informed decisions and optimise their operations.
Conclusion
Understanding the true meaning of predictive analytics and preventive maintenance is crucial for today’s solar industry. Leveraging its value will enable solar industry stakeholders to de-risk investments, maximise performance, and reduce costs. SmartHelio’s commitment to providing accurate and actionable insights sets us apart as a benchmark in the industry. Through early fault detection, optimised maintenance strategies, and reliable predictions, SmartHelio empowers decision-makers with data-driven approaches for a sustainable and efficient solar future.
Can AI alone solve solar maintenance challenges?
SmartHelio, a leading player in the SaaS solar industry, recently co-organized an informative webinar with PV Magazine titled “AI or not AI for fault prediction in solar plants: misconceptions and facts.” The webinar brought together industry experts and key partners of the company, Schneider Electric and VERBUND, to delve into their challenges, aspirations for scaling up, and how AI can support their objectives.
Throughout the event, SmartHelio shed light on the real-world problems faced by the industry and showcased how their innovative solution addresses issues such as data management, standardisation, and late fault identification. The discussions also explored the distinction between monitoring and analytics, as well as the limitations of AI algorithms. Moreover, the webinar highlighted the growing need for automation and predictive analytics to effectively manage expanding portfolios in the face of climate change.
In the dynamic landscape of the solar industry, achieving scalability and overcoming challenges is crucial. SmartHelio, an innovative solution, leverages the complementary strengths of artificial intelligence (AI), physics, and human expertise to revolutionise solar companies’ operations. By addressing issues like standardisation, data quality, availability, and reactive maintenance, SmartHelio empowers prominent solar companies at all levels, from asset management team to C-suite level, passing by performance and remote operations, to scale up their operations effectively.
Enhanced Data Quality and Standardisation: Fuelling Growth
With the integration of AI and physics, SmartHelio automates data collection and analysis, ensuring reliable and standardised information. This comprehensive approach guarantees high-quality data, enabling accurate performance assessments and informed decision-making. Standardisation across various solar assets provides invaluable insights and benchmarks for actionable improvements.
Proactive Maintenance: Eliminating Reactive Challenges
SmartHelio’s fusion of AI, physics, and human expertise allows for predictive and proactive maintenance strategies. By continuously monitoring key performance indicators, the system identifies anomalies and potential failures, enabling timely interventions. Proactive maintenance not only reduces downtime and operational costs but also extends the lifespan of solar assets, enhancing overall efficiency and profitability.
“Whereas physics-based models are very good on post failure analysis, with more Machine Learning knowledge in our data warehouse we will then be able to recognise very small deviations at an early stage to have prediction on where your next fault may probably happen”
– David Ebner, Project Engineer at VERBUND
Trust and Transparency: Foundations of Success
In an industry where trust and transparency are paramount, SmartHelio provides a transparent and auditable platform. This ensures data integrity and offers stakeholders full visibility into asset performance and operational metrics. Armed with trustworthy data, asset managers and performance managers can confidently make data-driven decisions, enhancing operational efficiency and driving business growth.
Client Testimonials: The Impact of SmartHelio
Leading solar industry players, including Schneider and VERBUND, have experienced remarkable potential transformations with SmartHelio. By seamlessly integrating AI and physics into their operations, these companies understand the possibility to streamline processes, reduce costs, and significantly increase productivity while opening the way to new business models.
“We have made an analysis on 12 months for a 3 year old, […] computed the lost revenues. And I can tell you that based on that, the energy manager at Schneider said, hey, we need to continue. So he was convinced that there was a value […] for more generation, safety and capability for new value for the future”
– Luc Hossenlopp, CTO Digitalisation at Schneider Electric
“SmartHelio was quickly identified as the best fit for us […] They identified all errors that we also found with our current monitoring solution. Plus, they also could identify some more failures which we didn’t know about yet.”
– David Ebner, Project Engineer at VERBUND
Autopilot: Full Control and Easy Integration
At the heart of SmartHelio lies its game-changing feature, Autopilot. This functionality empowers users with complete control over their solar assets, enabling automated actions and real-time optimisations. Moreover, Autopilot seamlessly integrates with existing software systems, ensuring a smooth collaboration between SmartHelio and other tools used by solar companies. This easy integration provides all teams and C-level people from solar companies with a comprehensive view of operations, saving time and enhancing productivity.
“Our system is built on three fundamental pillars: People, Physics, AI. Together, these pillars establish a foundation of trust, transparency and truth. This unique combination puts users in the center and give them full control over the results”
– Govinda Upadhyay, CEO & Founder of SmartHelio
Conclusion: Empowering the Solar Industry for Unparalleled Growth
SmartHelio’s integration of AI, physics, and human expertise offers a transformative solution for the solar industry. By addressing challenges related to lack of standardisation, data quality and availability issues, and reactive maintenance, SmartHelio enables leading solar companies to optimise operations, enable intelligent decision-making, and drive sustainable business growth. With Autopilot software providing full control and seamless integration thanks to its physics-AI unique combination, solar companies can unlock the true potential of their assets, maximising efficiency and profitability in an increasingly competitive landscape.
pv Magazine Webinar | Can AI alone solve solar maintenance challenges?
AI or not AI for fault prediction in solar plants: Misconceptions & Facts
SmartHelio co-organized an informative webinar with pv Magazine titled AI or not AI for fault prediction in solar plants: misconceptions and facts. The webinar brought together industry experts and key partners of the companies Schneider Electric and VERBUND to delve into their challenges, aspirations for scaling up, and how AI can support their objectives.
What to expect
- Innovative solutions addressing issues such as data management, standardization, and late fault identification
- Distinction between monitoring and analytics as well as the limitations of AI algorithms
- Need for automation and predictive analytics to effectively manage expanding portfolios in the face of climate change
Who is this webinar for?
- Asset managers
- O&M teams
- Investors & CXOs
- Solar data scientists
- Heads of innovation
- Directors of digitalization